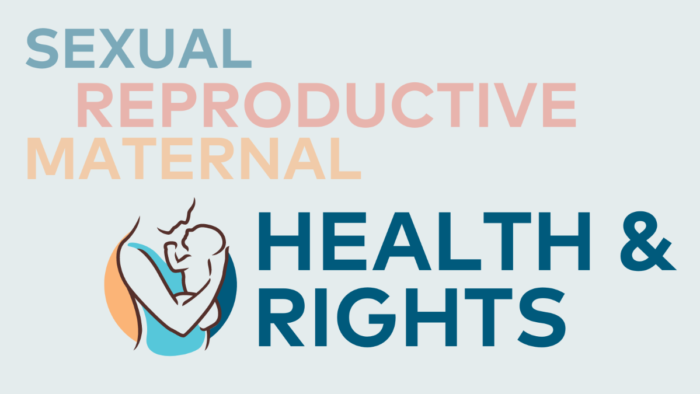
In 2023, Lacuna Fund launched a new call for proposals within our Health domain: Sexual, Reproductive, and Maternal Health & Rights. This call was made possible by Canada’s International Development Research Centre (IDRC).
Here are the awardees who have received funding to pursue projects within this focus. These teams will work to improve Sexual, Reproductive, and Maternal Health and Rights (SRMHR) outcomes in Africa and create datasets that will improve post-discharge care for mothers and babies, better label existing Pap smear data, and collect new data to better detect cervical cancer, identify and categorize mothers into low to high risk of birth complications during prenatal care, and more. In Africa, the Sexual, Reproductive, and Maternal Health and Rights of many are far from realized. We are excited for these Lacuna Fund grantees to develop open and accessible training and evaluation datasets for machine learning applications on SRMHR in Africa!
Smart Discharges for Mom & Baby: Building capacity for data-driven quality improvement in maternal-newborn care
Contact: Michael Kawooya ([email protected])
In 2020, 287,000 mothers died from complications of pregnancy or childbirth; one-third of these deaths (30%) occurred during tce first six weeks after birth (World Health Organization, 2023). Precision public health approaches leverage risk prediction to identify the most vulnerable patients and inform decisions around usicng scarce resources, including the frequency, intensity, and type of postnatal care follow-up visits.
However, these approaches may not accurately or precisely predict risk for specific sub-groups of women who are statistically underrepresented in the total population, such as women who experience stillbirths. With this project, the team aims to generate a synthetic dataset of sociodemographic and clinical variables and health outcomes for mother-newborn dyads in Uganda, using existing data collected during prior observational studies. They will use this dataset to enhance their risk prediction model for identifying women at a high risk of death or readmission in the six weeks after a hospital delivery to more accurately and precisely predict risk for mothers who experience stillbirths. They will work with research partners in Canada to train and mentor Ugandan data scientists in model development and data management and build local capacity in this field. The team will use their results to inform the development of a precision public health approach for improving post-discharge care that is inclusive of all mothers and newborns in partnership with the Ugandan Ministry of Health and other key stakeholders in maternal health.
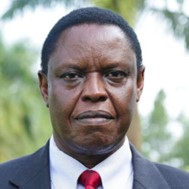
“This project builds upon our prior work to improve post-discharge care for children. This award from Lacuna Fund will help us overcome some of the most pressing challenges we face in providing high-quality healthcare to the people of Uganda. Firstly, it will help us build data-driven solutions to improve the transition from hospital to home for mothers and their newborns, which is critically needed to improve their survival. Secondly, it will help build health-focused data science capacity here in Uganda that will support this work and many other initiatives that are helping the vulnerable.” — Dr Nathan Kenya-Mugisha, Executive Medical Director, WALIMU
Dataset for Maternal Health Risks Stratification in Tanzania for Predictive and Responsible Machine Learning
Contact: Rukia Mwifunyi ([email protected])
Twitter: @mailab_io and @ECUREI Ug
This project aims to improve the early identification of pregnant women at risk of birth complications, a critical aspect of prenatal care for reducing adverse birth outcomes. This improvement will be realized through the implementation of Maternal Health Risks Stratification (MHRS), which involves categorizing pregnant women into different risk levels (e.g., high, medium, and low) based on a comprehensive analysis of both objective and subjective data derived from claims and laboratory test results. The goal is to enable referral hospitals to allocate resources more effectively by focusing on high-risk pregnancies while low-risk pregnancies are managed at community health centers. Therefore, the main objective is to create an open and inclusive dataset for MHRS for equitable and responsible machine-learning applications that can predict possible adverse birth outcomes in early pregnancy. This dataset will be compiled from pregnant women attending maternity health centers in underserved districts across various regions within Tanzania, where individualized maternal health data is currently scarce or not accessible to the public.
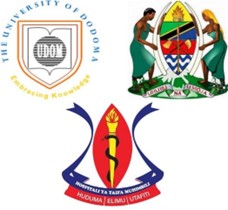
“In partnership with Muhimbili National Hospital (MNH), the Ministry of Health (MoH), and the Ministry of President’s Office, Regional Administration and Local Government (MoPO-RALG), the University of Dodoma (UDOM) will establish an inclusive and accessible dataset for Maternal Health Risks Stratification (MHRS). This initiative aims to facilitate responsible machine learning applications capable of predicting potential adverse birth outcomes during early pregnancy. To ensure representation and inclusion of marginalized communities, our project team will effectively gather and categorize data from expecting mothers attending maternity health centers for a minimum of nine months. We will focus on five underserved districts located in various regions across different zones in Tanzania. We are confident that this project will make a significant contribution to improving maternal health outcomes in Tanzania. We are excited about embarking on this project and express deep gratitude to Lacuna Fund for granting us with this exceptional opportunity.” — Rukia Mwifunyi, Principal Investigator, UDOM
The African Breast Imaging Dataset for Equitable Cancer Care (The ABreast Data)
Contact: Michael Kawooya ([email protected])
This study will introduce a community-based, data-driven breast care approach to close disparities in the early detection of breast cancer among black African women, who have the highest rates of premature deaths from breast cancer in the world. The team plans to curate a high-quality annotated breast imaging dataset of point-of-care ultrasound (POC-US) scans of 100 Ugandan and Nigerian women collected at regular intervals in the community. The dataset will be matched with mammograms collected at diagnostic imaging centers, biopsy pathological data, and comprehensive clinical profiles. This dataset will provide the first open and accessible training and evaluation breast imaging data for machine learning (ML) applications in Black African women and the first publicly available POC-US data in the world. More importantly, as low-cost imaging technologies such as POC-US and now POC magnetic resonance imaging (MRI), including the recent POC-MRI built in Uganda, show clinical promise, this dataset will enable the establishment of a framework for ML-guided community-based screening programs aimed at democratizing imaging services to increase access to high-value diagnostic care for vulnerable populations
They will achieve this by curating an inclusive imaging dataset matched with ground truth labels and use this dataset to develop an ML method for image segmentation/pathology identification for rapid diagnosis. The team’s expertise in community radiology, including training health workers to provide basic and frontline radiological services in rural and resource-limited settings, will be leveraged to establish the data collection framework at the community level. The step-by-step data collection workflow will include the following key features; at-home breast care self-examination; breast awareness, and community participatory research training; enrollment of study participants; imaging and clinical data collection. Together, the team, working with the community, will provide a high-value labeled ML dataset for POC-US that could make it easier to treat breast cancer, delay its onsets, or work toward prevention strategies for all breast cancer patients.
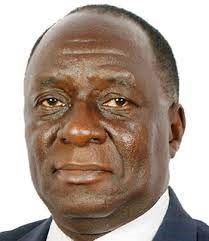
“The Ernest Cook Ultrasound Research and Education Institute (ECUREI) in partnership with The Medical Artificial Intelligence Laboratory (MAI Lab) at Crestview Radiology Inc, will establish a representative dataset for the development of imaging tools to significantly improve breast screening in Sub-Saharan Africa. This project provides a unique opportunity for the application of machine learning techniques to develop robust point-of-care imaging solutions that will bring advanced diagnostic imaging to the community, and to the most vulnerable at-risk groups – who are often unable to access such care. This joint effort builds on the team’s prior Lacuna-funded projects in the Health and Equity domain and will leverage protocols and methods for medical imaging data curation and annotation to ensure the success of this project. We are grateful for the continued support from Lacuna Fund to advance data science in Africa.” — Prof. Michael Kawooya – Project Leader, Ernest Cook Ultrasound Research and Education Institute (ECUREI)
Pap-Smear Microscopy data set for Cervical Cancer Screening
Contact: Rose Nakasi ([email protected])
Cervical cancer is a reproductive health burden among women, posing a significant public health challenge in Low- and Medium-Income Countries. To reduce the caseload and mortality rate associated with cervical cancer, reliable and early screening and diagnosis of the disease is essential. Pap smear using microscopy in screening for cervical cancer is a central component of current WHO recommendations. In Uganda and Sub-Saharan Africa, the utilization of Pap smear microscopy is challenged by insufficient expertise to interpret the images, affecting the efficiency, effectiveness, and accuracy of cervical cancer screening. The project team aims to curate a digital dataset from existing Pap smear image slides using their already developed data collection apparatus, comprised of a mobile smartphone attached to the eyepiece of a microscope. This localized and labeled dataset will be used for real-world automatic cervical cancer screening in Uganda. In collaboration with microscopists, pathologists, and cancer cytology experts at the Mulago referral hospital, this study will deliver on a labeled Pap-smear image dataset of 1,000 images. The datasets will be annotated in formats that deliver on a wide range of machine learning use cases, including object detection, classification, and segmentation tasks. They expect the image dataset will be used for effective cervical cancer detection and improved reproductive health among women in Sub-Saharan Africa.
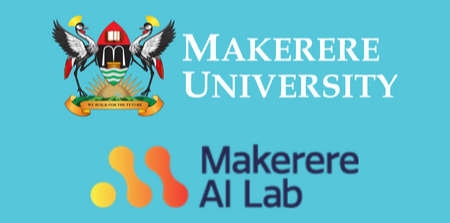
“With support from Lacuna fund, a localized and labeled pap-smear microscopy dataset will be curated for real-world automatic cervical cancer screening towards improved reproductive health among women in Uganda.” — Dr. Rose Nakasi, Principal Investigator